Entropy
Entropy is a heuristic that measures the model’s uncertainty about the class of the image. The higher is entropy – the more “confused” the model is. As a result, data samples with higher entropy are ranked higher and offered for annotation.
Below is a mathematical formula for entropy calculation:

Imagine that our model predicts whether an object is a dog or a muffin. Let’s say it gave the following probabilities for instances A and B:
- Instance A: “dog” – 0.5, “muffin” – 0.5.
Entropy = H([0.5,0.5]) = 1.0 - Instance B: “dog” – 1.0, “muffin” – 0.0.
Entropy = H([1.0,0.0]) = 0.0
As you see, the model was completely confused when the class probabilities were equal. In contrast, when one of the classes had a 100% probability, the entropy was equal to 0 since there was no uncertainty.
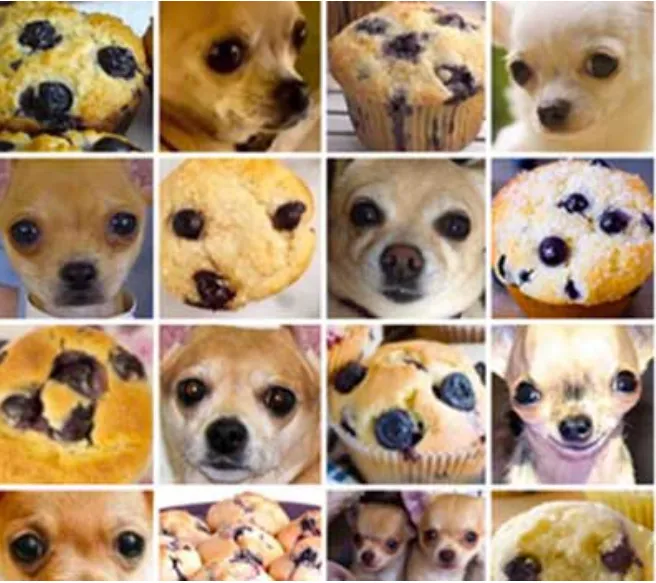
Entropy might be useful in datasets with very diverse images and classes.
Learn more about the other heuristics: