Overfitting
When building a Machine Learning solution, you might end up with a model that does really well on training but shows a significant performance decrease when applied to the production data. Data Scientists face such a challenge regularly and call it overfitting.
In Computer Vision, such a problem is not a rare occurrence. This makes overfitting a sworn enemy one needs to be able to detect and overcome or even prevent to have a chance to build a successful model.
On this page, we will:
Define the overfitting term;
Explore the bias-variance tradeoff in Machine Learning;
Come up with some simple overfitting examples;
Understand the potential reasons behind a model overfitting;
Learn how to detect overfitting early on;
And explore more than 10 ways of preventing and overcoming overfitting issues.
Let’s jump in.
What is Overfitting?
To define the term, overfitting is such a Machine Learning model behavior when the model is very successful in training but fails to generalize predictions to the new, unseen data. In other words, the model shows a high ML metric during training, but in production, the metric is significantly lower.
Overfitting is not a desirable model behavior as an overfitted model is not robust or trustworthy in a real-world setting, undermining the whole training point.
Let’s take a look at overfitting on a deeper level.
Bias-Variance tradeoff
The key to understanding overfitting lies in the bias-variance tradeoff concept. As you might know, when training an ML algorithm, developers minimize its loss, which can be decomposed into three parts: noise (sigma), bias, and variance.
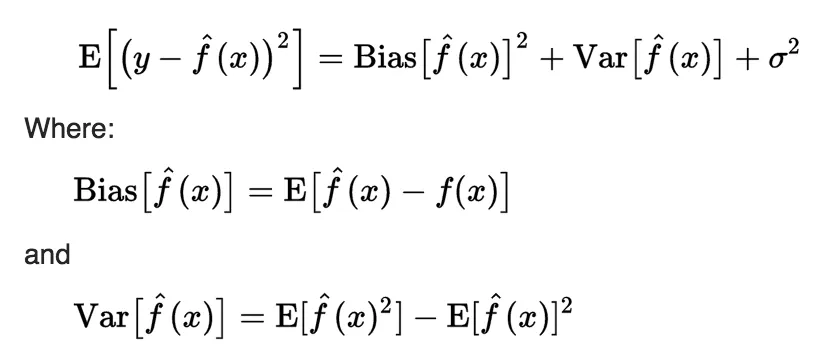
Source
Let’s get through them one by one:
The first component describes the noise in the data and is equal to the error of the ideal algorithm. There will always be noise in the data because of the shift from the training samples to real-world data. Therefore, it is impossible to construct an algorithm with less error;
The second component is the bias of the model. Bias is the deviation of the average output of the trained algorithm from the prediction of the ideal algorithm;
The third component is the variance of the model. Variance is the scatter of the predictions of the trained algorithm relative to the average prediction.
The bias shows how well you can approximate the ideal model using the current algorithm. The bias is generally low for complex models like trees, whereas the bias is significant for simple models like linear classifiers. The variance indicates the degree of prediction fluctuation the trained algorithm might have depending on the data it was trained on. In other words, the variance characterizes the sensitivity of an algorithm to changes in the data. As a rule, simple models have a low variance,
and complex algorithms - a high one.
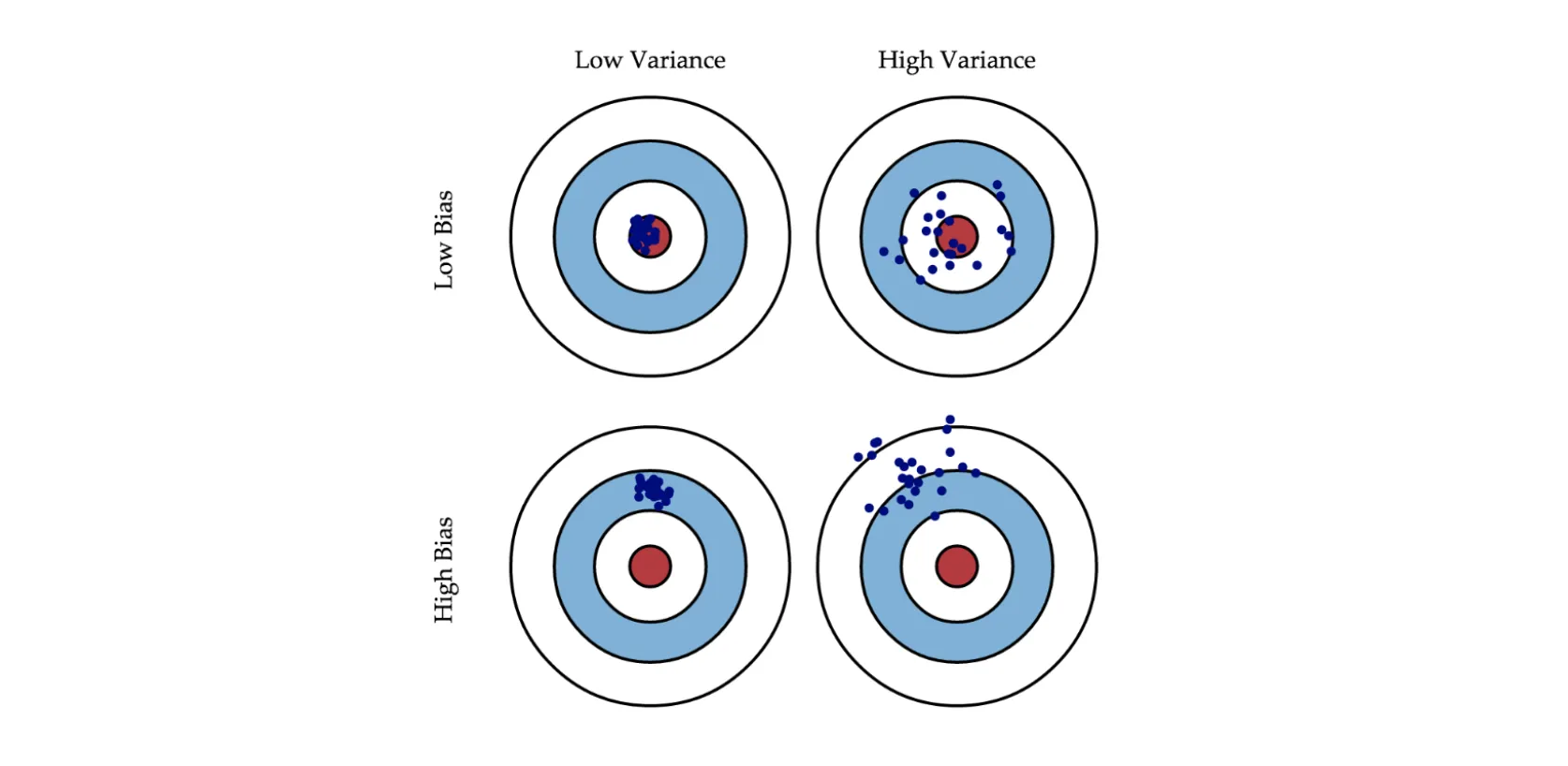
Source
The picture above shows models with different biases and variances. A blue dot represents each model, so one dot corresponds to one model trained on one of the possible training sets. Each circle characterizes the quality of the model - the closer to the center, the fewer the model's error on the test set.
As you can see, having a high bias means that the model's predictions will be far from the center, which is logical given the bias definition. With variance, it is trickier as a model can fall both relatively close to the center as well as in an area with large error.
Bias and variance have an inverse relation: when bias is high, variance is low, and vice versa. This is well reflected in the image below.
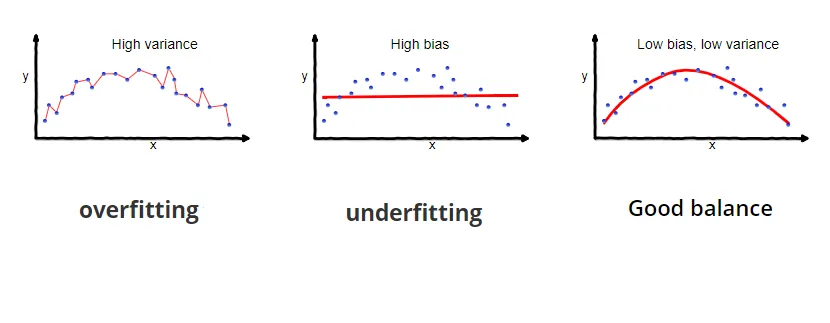
Source
Thus, overfitting is such a scenario when the bias is so low that the model almost does not make any mistakes, and the variance is so high that the model can predict samples far from the average.
Overfitting examples
Let’s draw some simple overfitting examples.
Imagine solving a Computer Vision Classification task identifying whether there is a dog on an image. Unfortunately, you train a model on a dataset that features dogs in a park laying on the grass or playing in it. Surprisingly, once you start testing your model, showing it the pictures of dogs in other circumstances, you see a significant performance decrease. This is because your model overfitted and probably learned to recognize grass and use it as a feature for Classification.
Another case is about credit scoring. You solve a binary Classification task and try to predict whether an applicant will return the money. Your model shows a very high accuracy both on train and test sets but starts acting weird in production, accepting every credit application. This is because, in the initial training set, you had a significant class imbalance towards the people who returned the money. The model overfitted and learned that the best policy to obtaining high metric values is to accept every credit application as you have little to no negative cases in your train set.
Why does overfitting happen?
Overfitting reasons may vary from use case to use case. However, in general, you might want to check the following points.
Overfitting reason: Little and repetitive dataset and class imbalance
If your dataset is small or repetitive and lacks class, condition, lighting, background, etc. variety, your model will be naturally limited by the seen examples and fail to extrapolate its predictive power to more complex real-life cases.
Overfitting reason: Very complex Machine Learning model
Modern ML models have many parameters in them - the number might be surreal. The more parameters your model has, the more flexible it is regarding training. In simple words, the model might be too smart for the use case, memorizing complex patterns from the training set instead of finding more general patterns.
In the worst-case scenario, your model will memorize the whole training set without coming close to the general pattern.
For example, neural networks might have millions of parameters, making them prone to overfitting. In comparison, simple models such as linear regression are a bit more robust but have trouble dealing with complex, high-dimensional data or capturing non-linear relationships.
Overfitting reason: Endless training
It might seem like an excellent idea to train a model for a significant amount of time, epochs, or iterations. More training - better results. Well, it’s not how it works in Machine Learning.
If a model gets trained for an extended amount of time and batches, at some point, it will inevitably start learning non-essential features - noise, which is a straight path to overfitting.
How to detect overfitting?
There are different approaches to overfit model detection. You can do it by checking the learning curves, empirically, or through cross-validation. Let’s check these methods one by one.
Overfitting detection: Learning curves
To diagnose the overfit, you can take a look at the model’s learning curves - the plot that reflects the model’s loss on the train and test data over iterations.
At some point, you might see that the training loss starts approaching zero while the validation loss suddenly rises. This is precisely where the model stopped extracting the general pattern and started overfitting.
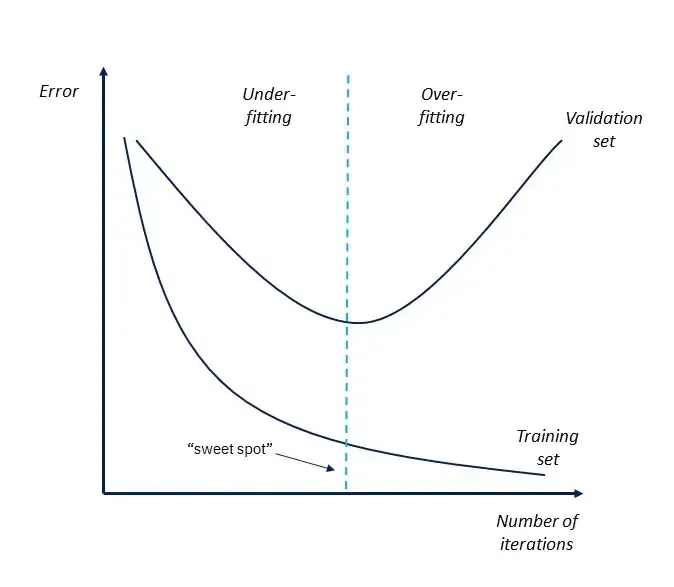
Source
Overfitting detection: Empirical heuristic
An empiric way of detecting overfitting is by comparing the Machine Learning metrics on train and test steps. On training, the model will show nearly perfect predictions, making the metric sky-high. However, the model’s predictions will be inaccurate on unseen data, leading to notable failure in the metric’s value (we mean tens of percent decrease).
Overfitting detection: Cross-Validation
The most accurate approach to detecting overfitting (and other model weaknesses) is k-fold cross-validation. The algorithm is the following:
Shuffle your dataset and split it into k equal-sized folds;
Train your model on k - 1 folds and test its performance on the left-out fold;
Repeat the procedure k times so that each fold is used as a validation set once;
Take the average across the model’s performance on all the folds and analyze the obtained value.
How to prevent and overcome overfitting?
Overfitting is a sworn enemy of every Data Scientist, but over time, developers have come up with valuable techniques to prevent and overcome overfitting in Machine Learning models and neural networks.
Some of these approaches are complex, so on this page, we will only draw a brief description of each method and leave links to more in-depth pages exploring a specific way.
For now, the most common techniques for dealing with overfitting are:
Regularization techniques. Regularization introduces additional terms in the loss function that punish a model for having high weights. Such an approach reduces the impact of individual features and forces an algorithm to learn more general trends. Besides traditional regularization techniques such as L1 and L2, in neural networks, weight decay and adding noise to inputs can also be applied for regularization purposes;
Early stopping. Early stopping is a way that closely monitors the validation loss curve while training and stops the training process as soon as the validation loss stops improving. Such a method protects the model and does not allow it to learn noise in the data, thus making it less complex;
Dropout. Dropout is one of the primary approaches to dealing with overfitting in neural networks. This layer randomly deactivates some neurons during each training iteration, creating an ensemble of smaller networks. As a result, the network learns not to rely on specific neurons but rather to pick up on generalized patterns;
Data Augmentation. Data Augmentation suggests prompting the model to be more robust to noise by extending the training set with augmented training samples. For example, you can rotate existing images, change their color palette, or apply various other transformations. This is especially something to keep your eye on if you have a small or not very diverse dataset;
Batch Normalization. Batch Normalization brings normalized activations within each neural network layer on training. Although this technique was not initially intended to prevent overfitting, it still has a nice regularization side-effect, making training more stable and mitigating overfitting;
Reducing the complexity. As mentioned above, a model might overfit because it is too complex. You can simplify its architecture to try and overcome this;
Ensembling. Ensembling suggests combining predictions of several models to formulate the final output. It is proven to help improve the solution’s generalization capabilities and reduce the overfitting risks. By the way, by applying Dropout, you use the ensembling concept to the neural network;
And many more. Plenty of less popular methods still might be the perfect fit for your case. For example, you can exclude irrelevant features from your data - Feature Selection, collect more diverse data to address edge cases or class imbalance, work with extensive model’s nodes, connections, and parameters after training - Pruning, or take an alternative approach and run numerous experiments with various hyperparameters to find the balance between the model complexity and generalization.
These approaches provide a wide range of techniques to address overfitting issues and ensure better generalization capabilities of a model. The exact choice of a method depends significantly on the use case, data, model, goals, etc. Please explore the field before opting for a certain way.
Key takeaways: Overfitting
Overfitting is such a Machine Learning model behavior when the model successfully trains but fails to generalize predictions to the new, unseen data.
The most accurate approach to detecting overfitting is k-fold cross-validation.
The general advice we can give you is to remember that overfitting exists but techniques for overcoming it are there as well. Follow the simple five steps listed below to ease your life from overfitting when developing a Machine Learning solution:
Try to collect as diverse, extensive, and balanced a dataset as possible;
Keep track of the model learning curves to detect overfitting early on;
Do not use too complex models without the need for that;
Apply regularization techniques;
Always validate your model performance on a set of examples not seen during training (for instance, using cross-validation).
These steps will not guarantee getting rid of overfitting for good, as there remains a discrepancy between your training set and real-world data. However, it is your responsibility and interest to make your model as reliable, robust, and generalizable as possible.